After ten years, Crohn’s disease, a chronic inflammatory gastrointestinal condition, is expected to have a 40% postoperative symptomatic recurrence rate. Although there are scoring techniques for measuring Crohn’s disease activity and postoperative recurrence, there is no scoring system for predicting whether Crohn’s disease will return.
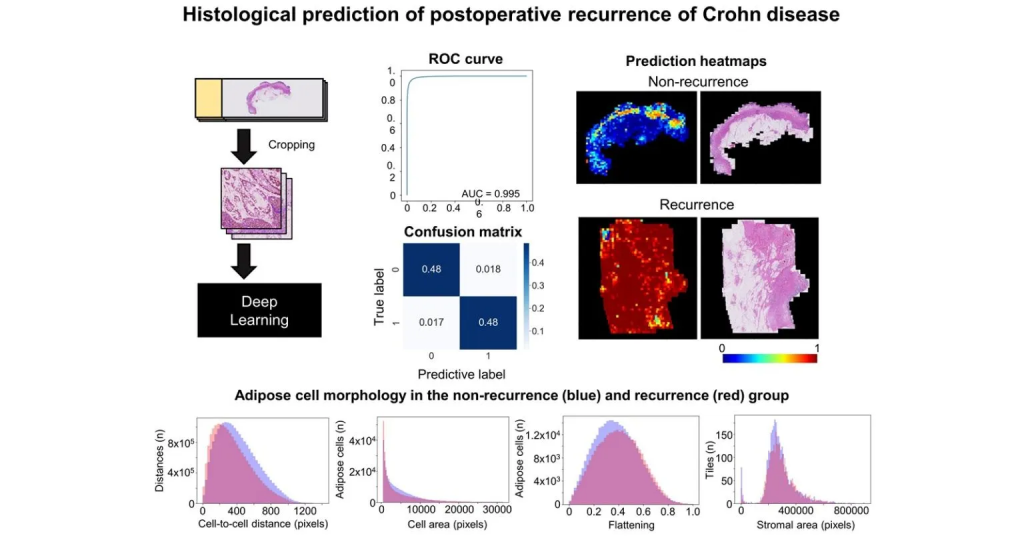
Takahiro Matsui, MD, Ph.D., and Eiichi Morii, MD, Ph.D., of the Department of Pathology, Osaka University Graduate School of Medicine, Osaka, Japan, explained that “much of the analysis of histopathological pictures utilizing AI in the past has targeted on malignant tumors. “By analyzing histopathology photos with AI, we aimed to gain therapeutically useful information for a larger range of disorders.” We concentrated on Crohn’s disease, which has a high rate of postoperative recurrence.”
Between January 2007 and July 2018, 68 Crohn’s disease patients had intestinal resection for the study. They were divided into two groups based on whether or not they had a recurrence of the condition within two years after the surgery. Each group was split into two subgroups, one for training and the other for AI model validation. For training, whole slide pictures of surgical specimens were cropped into tile images, annotated for the presence or absence of postsurgical recurrence, and then processed using EfficientNet-b5, a commercially available AI model for image classification. When the deep learning model was tested using unlabeled photographs, the results showed that it correctly identified the unlabeled images based on the presence or absence of the disease occurrence.
Then, to select areas and histological features from which the machine learning algorithm accurately predicts recurrence, prediction heat maps were created. The images depicted all layers of the intestinal wall. The machine learning algorithm correctly predicted the subserosal adipose tissue layer, according to the heatmaps. On the other hand, the model was less accurate in some areas, such as the mucosal and correct muscle layers. The non-recurrence and recurrence test datasets were used to choose the images with the most accurate predictions. Adipose tissue was present in all images with the best predictive results.